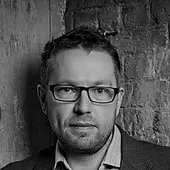
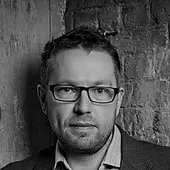
Ensuring data quality in market research is critical to deriving meaningful and actionable insights. Poor data quality can lead to erroneous conclusions, misinformed decisions, and ultimately wasted resources. Here are several key strategies to ensure data quality in market research:
Clear research objectives
Establishing clear and precise research objectives is the foundation of high-quality data. Well-defined objectives guide the research design, data collection methods, and analysis procedures. They help ensure that the data collected is relevant and aligned with the goals of the study.
Robust research design
A robust research design encompasses the following elements:
- Sampling: Choose an appropriate sampling method (e.g., random, stratified, or cluster sampling) to ensure the sample is representative of the target population.
- Collection techniques: Develop reliable and valid data collection techniques, such as surveys or questionnaires. Use pre-tested and standardized instruments whenever possible.
- Pilot Testing: Conduct pilot testing to identify potential issues with the research design or data collection instruments. This helps in refining the tools and methods before the main study.
Data collection methods
Selecting appropriate data collection methods is crucial for data quality. Common methods include surveys, interviews, focus groups, and observations. Each method has its strengths and weaknesses, and the choice should be aligned with the research objectives and the nature of the data required. Ensure that the data collection process is consistent and that the data collectors are well-trained.
Data management and cleaning
Data management involves organizing, storing, and maintaining data in a way that ensures its integrity and accessibility. Key practices include:
- Data Cleaning: Regularly check for and correct errors, such as duplicate entries, missing values, and outliers. Use automated tools and manual checks to ensure data accuracy.
- Data Documentation: Maintain detailed documentation of data sources, collection methods, and any transformations applied to the data. This ensures transparency and reproducibility.
Quality control procedures
Implementing quality control procedures throughout the research process helps identify and address issues as they arise. Some key procedures include:
- Validation Checks: Use validation checks to ensure data consistency and accuracy. For example, cross-verify data with external sources or use logical checks to identify inconsistencies.
- Training and Supervision: Provide comprehensive training to data collectors and supervisors. Regularly monitor their work to ensure adherence to protocols and standards.
Ethical considerations
Ethical considerations play an essential role in ensuring data quality. Obtain informed consent from participants, protect their privacy, and ensure data confidentiality. Ethical practices build trust with participants, leading to more honest and accurate responses.
Analytical rigor
Apply rigorous analytical techniques to process and analyze the data. Use appropriate statistical methods and software tools to ensure accurate and reliable results. Avoid common pitfalls such as overfitting models, ignoring bias, or misinterpreting correlations as causations.
Continuous improvement
Data quality is an ongoing concern, and continuous improvement practices help maintain high standards. Regularly review and update research methodologies, data collection instruments, and quality control procedures. Learn from past research projects to identify areas for improvement.
Conclusion
Ensuring data quality in market research is a multifaceted process that involves careful planning, execution, and ongoing evaluation. By establishing clear objectives, employing robust research design, selecting appropriate data collection methods, maintaining rigorous data management practices, implementing quality control procedures, adhering to ethical standards, and applying analytical rigor, researchers can enhance the reliability and validity of their data. Continuous improvement efforts help sustain data quality over time, ultimately leading to more accurate and actionable insights that drive better decision-making.